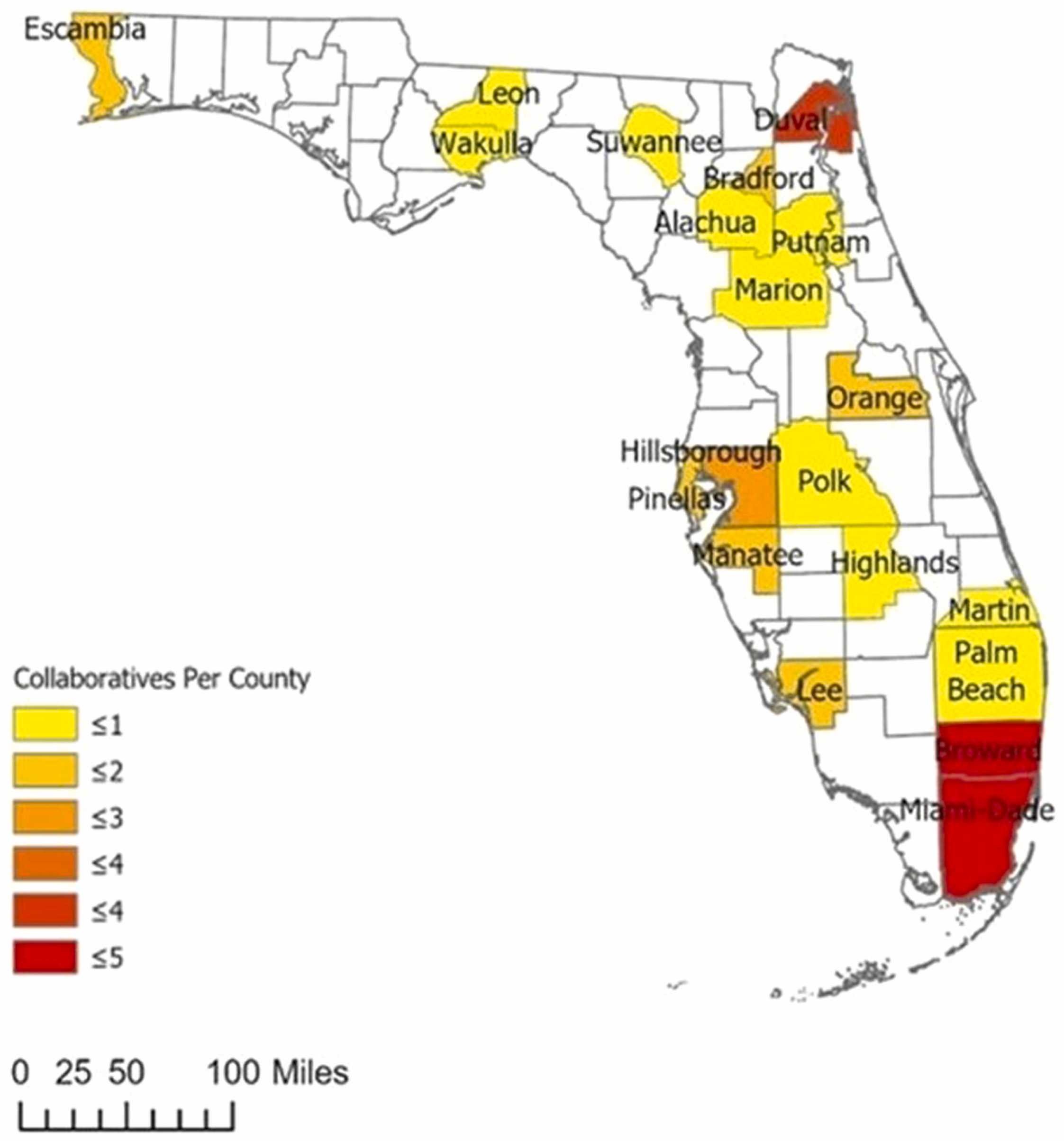
New article first published online: Social Networks
ABSTRACT: Interorganizational coalitions or collaboratives in healthcare are essential to address the health challenges of local communities, particularly during crises such as the Covid-19 pandemic. However, few studies use large-scale data to systematically assess the network structure of these collaboratives and understand their potential to be resilient or fragment in the face of structural changes. This paper analyzes data collected in 2009–2017 about 817 organizations (nodes) in 42 healthcare collaboratives (networks) throughout Florida, the third-largest U.S. state by population, including information about interorganizational ties and organizations’ resource contributions to their coalitions. Social network methods are used to characterize the resilience of these collaboratives, including identification of key players through various centrality metrics, analyses of fragmentation centrality and core/periphery structure, and Exponential Random Graph Models to examine how resource contributions facilitate interorganizational ties. Results show that the most significant resource contributions are made by key players identified through fragmentation centrality and by members of the network core. Departure or removal of these organizations would both strongly disrupt network structure and sever essential resource contributions, undermining the overall resilience of a collaborative. Furthermore, one-third of collaboratives are highly susceptible to disruption if any fragmentation-central organization is removed. More fragmented networks are also associated with poorer health-system outcomes in domains such as education, health policy, and services. ERGMs reveal that two types of resource contributions – community connections and in-kind resource sharing – are especially important to facilitate the formation of interorganizational ties in these coalitions.